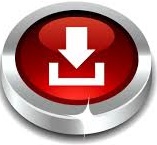
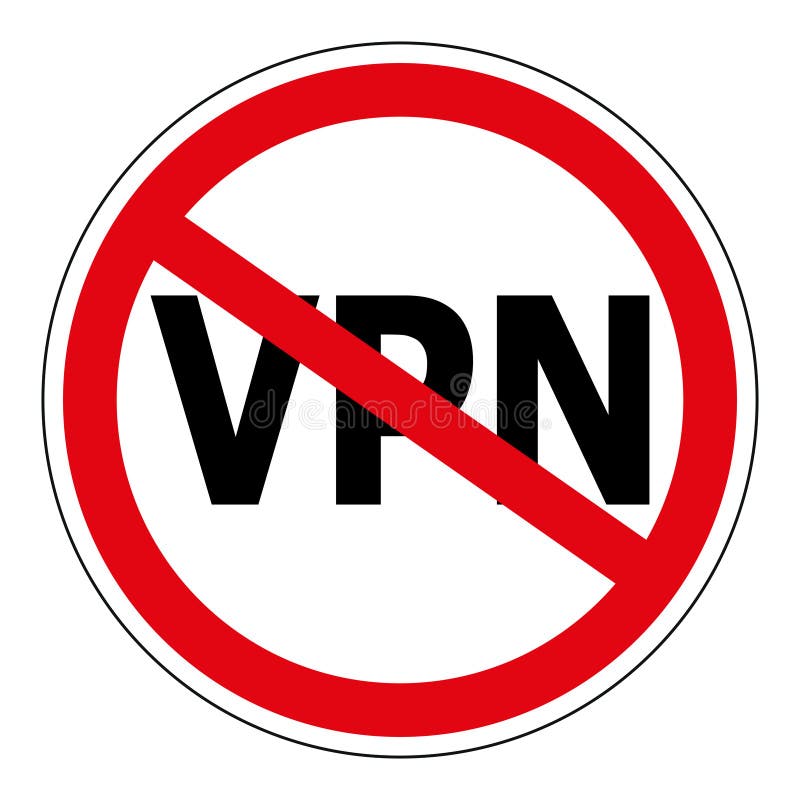
Worked on learning of efficient low resolution video transforms to classify actions from extreme low resolution videos. There can also be hidden backdoors installed by the manufacturer or the government, guaranteeing their access to cameras at one’s home. In the worst case, the users are under the risk of being monitored by a hacker if their cameras or robots at home are cracked. All these create a potential risk of one’s private videos being snatched by someone else. They sometimes even require network access to high computing power servers, sending potentially privacy-sensitive images/videos. Most computer vision algorithms require loading high resolution images/videos (that contain privacy-sensitive data) to CPU/GPU memory to enable visual recognition. On one hand, we want the camera systems/robots to recognize important events and assist human daily life by understanding its videos, but on the other hand we also want to ensure that they do not intrude the user’s or others’ privacy. Simultaneously, there is an increasing concern in these systems invading the privacy of their users in particular, from unwanted video taking and its sharing. In this paper, our goal is to create such a system. Ideally, we would like a face anonymizer that can preserve Alex’s privacy (i.e., make his face no longer recognizable as Alex) while at the same time unaltering his actions. However, you do not want your personal assistant to record Alex’s face, because you are concerned about his privacy information since the camera could potentially be hacked. Figure 1: Imagine the following scenario: you would like a personal assistant that can alert you when your adorable child Alex performs undesirable actions, such as eating mom’s make-up or drinking dirty water out of curiosity. For instance, cities are adopting networked camera systems for policing and intelligent resource allocation, individuals are recording their lives using wearable devices, and service robots at homes and public places are becoming increasingly available and popular.
#Sign up anonymizer universal full
READ FULL TEXT VIEW PDFĬomputer vision technology is enabling automated understanding of large-scale visual data, making it crucial for many societal applications with ubiquitous cameras. We experimentally confirm theīenefit of our approach compared to conventional hand-crafted video/ faceĪnonymization methods including masking, blurring, and noise adding. Minimal effect on action detection performance.
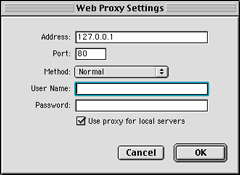
That performs a pixel-level modification to anonymize each person's face, with Performance, and (2) a discriminator that tries to extract privacy-sensitive

Human face) while still trying to maximize spatial action detection That modifies the original video to remove privacy-sensitive information (i.e., Training setting in which two competing systems fight: (1) a video anonymizer Principled approach for learning a video face anonymizer. On one hand, we want theĬamera systems/robots to recognize important events and assist human daily lifeīy understanding its videos, but on the other hand we also want to ensure that Privacy of their users by recording unwanted videos. Making intentional adjustments to the data with the purpose of hiding it from the customer would be considered a breach of GDPR.There is an increasing concern in computer vision devices invading the However, all of these changes must only be the natural ones which would have happened regardless of whether the request was made. It is completely fine that the data changed since the time of the request. The answer is that you should send to the customer the most up-to-date version of their data at the time of complying with their request. You might be asking then which version of the data are you supposed to provide. This means that some of the customer's data will be deleted. You have had probably set specific retention periods for your events. New customer data will, therefore, be added and some will be rewritten. The customer usually continues their activity on your website even after making the request. The data will probably change in these two ways: It is very likely that personal data will change between the time a customer makes a request and you complying with it. Real-time Customer Segments for Discovery.
#Sign up anonymizer universal how to
How to setup pre-built templates in Bloomreach Engagement.Product Recommendations in Bloomreach Engagement.Two-way messaging and Keywords Auto Responses.
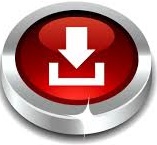